Light Field Denoising by Sparse 5D Transform Domain Collaborative Filtering
3rd August 2017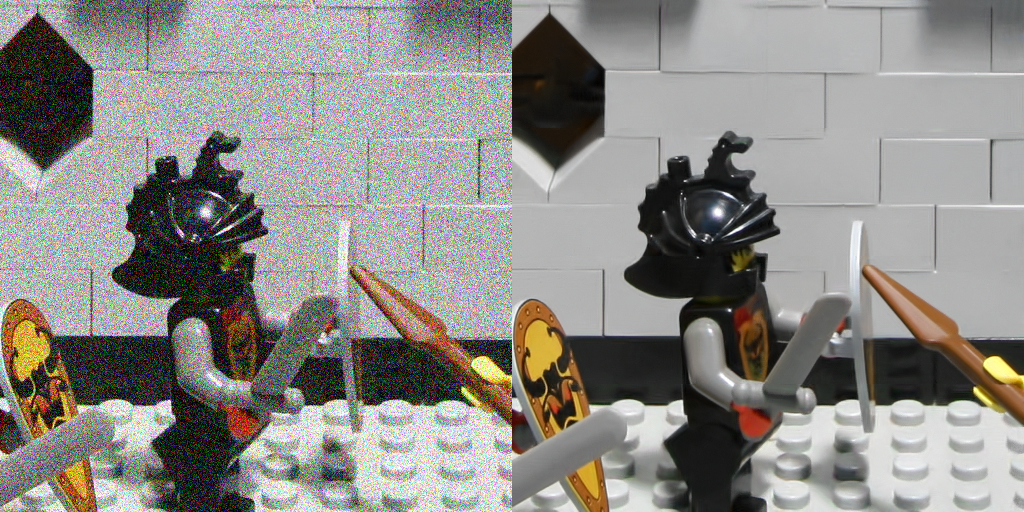
In this paper, we propose to extend the state-of-the-art BM3D image denoising filter to light fields, and we denote our method LFBM5D. We take full advantage of the 4D nature of light fields by creating disparity compensated 4D patches which are then stacked together with similar 4D patches along a 5th dimension. We then filter these 5D patches in the 5D transform domain, obtained by cascading a 2D spatial transform, a 2D angular transform, and a 1D transform applied along the similarities. Furthermore, we propose to use the shape-adaptive DCT as the 2D angular transform to be robust to occlusions.
Results show a significant improvement in synthetic noise removal compared to state-of-the-art methods, for both light fields captured with a lenslet camera or a gantry.
Experiments on Lytro Illum camera noise removal also demonstrate a clear improvement of the light field quality.
This paper was given a Top 10% Paper Award at the MMSP 2017 conference held in Luton, UK.
For the super-resolution extension SR-LFBM5D, see this page.
Implementation
The C/C++ source code is available on github.
Related publications
Additional results
Visual results complementing the paper are shown below.
We then show in Tables 1 and 2 the average results presented in the paper. The corresponding detailed results are shown in Table 3 and 4.
Visual results
We show in videos below (click on an image to start a video) side by side comparisons of noisy and de-noised light fields for different noise levels (? corresponds to the white gaussian noise standard deviation). On the top left corner of each video is highlighted the sub-aperture image being displayed. Note that some videos may exhibit encoding artifacts.
?=10 | ?=30 | ?=50 | |
Lego Knights | ![]() |
![]() |
![]() |
Amethyst | ![]() |
![]() |
![]() |
Tarot Cards and Crystal Ball (Small Angle) | ![]() |
![]() |
![]() |
Bikes | ![]() |
![]() |
![]() |
Magnets 1 | ![]() |
![]() |
![]() |
Vespa | ![]() |
![]() |
![]() |
Below, we show results for lenslet camera (Lytro Illum) noise removal.
Color Chart 1 | Desktop | ISO Chart 12 | Magnets 1 |
![]() |
![]() |
![]() |
![]() |
Average PSNR results
The ?PSNR lines in Tables 1 and 2 correspond to the PSNR gap between the proposed approach and the best state-of-the-art method. Values highlighted in bold correspond to the best performing method for a given noise level.
?=10 | ?=20 | ?=30 | ?=40 | ?=50 | |
HF4D | 31.070 | 25.798 | 22.607 | 20.338 | 18.586 |
BM3D | 35.421 | 32.852 | 31.357 | 30.247 | 29.321 |
BM3D EPI | 36.088 | 33.476 | 31.905 | 30.712 | 29.671 |
VBM4D | 36.075 | 33.522 | 31.923 | 30.674 | 29.630 |
VBM4D EPI | 36.129 | 33.510 | 31.925 | 30.719 | 29.721 |
LFMB5D 1st step | 34.388 | 32.810 | 31.684 | 30.743 | 29.911 |
LFMB5D 2nd step | 36.503 | 34.214 | 32.868 | 31.843 | 30.987 |
?PSNR | 0.374 | 0.692 | 0.943 | 1.124 | 1.266 |
?=10 | ?=20 | ?=30 | ?=40 | ?=50 | |
HF4D | 30.577 | 25.432 | 22.213 | 19.921 | 18.164 |
BM3D | 38.805 | 35.268 | 33.126 | 31.560 | 30.267 |
BM3D EPI | 38.895 | 35.645 | 33.489 | 31.896 | 30.594 |
VBM4D | 39.269 | 35.588 | 33.212 | 31.436 | 30.019 |
VBM4D EPI | 38.697 | 35.604 | 33.558 | 32.026 | 30.809 |
LFMB5D 1st step | 39.340 | 35.817 | 33.377 | 31.496 | 29.971 |
LFMB5D 2nd step | 40.389 | 37.772 | 36.031 | 34.6713 | 33.511 |
?PSNR | 1.120 | 2.127 | 2.473 | 2.645 | 2.701 |
Detailed PSNR results
Ankylosorus & Dipplodocus 1 | Bikes | Color Chart 1 | Danger de Mort | Desktop | Flowers | Fountain & Vincent 2 | Friends 1 | ISO Chart 12 | Magnets 1 | Stone Pillar Outside | Vespa | |
HF4D | ||||||||||||
?=10 | 30.881 | 30.940 | 31.038 | 31.460 | 31.160 | 31.302 | 30.791 | 30.987 | 30.901 | 30.943 | 30.973 | 31.463 |
?=20 | 25.383 | 25.622 | 25.957 | 26.081 | 26.196 | 26.071 | 25.654 | 25.933 | 25.478 | 25.393 | 25.688 | 26.116 |
?=30 | 22.047 | 22.459 | 22.775 | 22.953 | 23.072 | 22.965 | 22.478 | 22.832 | 22.139 | 22.006 | 22.556 | 22.999 |
?=40 | 19.672 | 20.247 | 20.489 | 20.758 | 20.816 | 20.766 | 20.200 | 20.614 | 19.756 | 19.583 | 20.345 | 20.810 |
?=50 | 17.846 | 18.553 | 18.715 | 19.069 | 19.063 | 19.064 | 18.426 | 18.890 | 17.925 | 17.721 | 18.634 | 19.121 |
BM3D | ||||||||||||
?=10 | 36.094 | 34.760 | 35.711 | 35.039 | 35.706 | 34.250 | 34.308 | 35.518 | 34.948 | 36.044 | 33.928 | 38.750 |
?=20 | 34.604 | 31.834 | 33.640 | 32.082 | 33.034 | 30.996 | 31.373 | 32.804 | 32.537 | 34.485 | 30.870 | 36.310 |
?=30 | 33.661 | 30.175 | 32.426 | 30.368 | 31.528 | 29.182 | 29.734 | 31.231 | 31.027 | 33.405 | 29.319 | 34.226 |
?=40 | 32.865 | 28.968 | 31.456 | 29.136 | 30.432 | 27.910 | 28.559 | 30.083 | 29.876 | 32.452 | 28.310 | 32.919 |
?=50 | 32.140 | 27.939 | 30.606 | 28.140 | 29.531 | 26.893 | 27.574 | 29.135 | 28.912 | 31.564 | 27.565 | 31.849 |
BM3D EPI | ||||||||||||
?=10 | 36.131 | 35.589 | 34.977 | 36.097 | 37.061 | 35.831 | 34.637 | 36.763 | 35.065 | 36.286 | 35.284 | 39.332 |
?=20 | 34.754 | 32.635 | 32.726 | 33.077 | 33.894 | 32.534 | 31.818 | 34.141 | 32.440 | 34.881 | 32.280 | 36.532 |
?=30 | 33.853 | 30.979 | 31.256 | 31.275 | 31.903 | 30.607 | 30.199 | 32.494 | 30.966 | 33.921 | 30.765 | 34.638 |
?=40 | 32.951 | 29.783 | 30.126 | 29.839 | 30.781 | 29.188 | 29.032 | 31.192 | 29.963 | 32.937 | 29.658 | 33.094 |
?=50 | 32.032 | 28.779 | 29.102 | 28.542 | 29.866 | 27.992 | 28.126 | 30.079 | 29.219 | 31.936 | 28.637 | 31.746 |
VBM4D | ||||||||||||
?=10 | 35.966 | 35.628 | 35.401 | 36.008 | 36.464 | 35.967 | 34.742 | 36.631 | 35.326 | 36.101 | 35.467 | 39.195 |
?=20 | 34.219 | 32.772 | 33.302 | 33.049 | 33.869 | 32.776 | 32.104 | 33.914 | 33.040 | 34.421 | 32.483 | 36.310 |
?=30 | 33.036 | 31.065 | 32.014 | 31.266 | 32.242 | 30.840 | 30.557 | 32.241 | 31.549 | 33.190 | 30.714 | 34.358 |
?=40 | 32.013 | 29.792 | 30.947 | 29.941 | 30.965 | 29.406 | 29.391 | 30.965 | 30.350 | 32.103 | 29.388 | 32.831 |
?=50 | 31.074 | 28.762 | 29.999 | 28.868 | 29.891 | 28.282 | 28.430 | 29.900 | 29.316 | 31.117 | 28.327 | 31.592 |
VBM4D EPI | ||||||||||||
?=10 | 36.374 | 35.692 | 35.515 | 36.059 | 36.467 | 35.648 | 34.638 | 36.685 | 35.334 | 36.326 | 35.537 | 39.271 |
?=20 | 34.303 | 32.865 | 33.081 | 33.175 | 33.860 | 32.708 | 31.892 | 34.057 | 32.885 | 34.330 | 32.589 | 36.376 |
?=30 | 33.025 | 31.185 | 31.618 | 31.446 | 32.317 | 30.966 | 30.300 | 32.445 | 31.391 | 33.058 | 30.918 | 34.433 |
?=40 | 31.978 | 29.951 | 30.467 | 30.172 | 31.131 | 29.689 | 29.119 | 31.225 | 30.242 | 32.006 | 29.721 | 32.927 |
?=50 | 31.045 | 28.960 | 29.502 | 29.145 | 30.129 | 28.678 | 28.161 | 30.203 | 29.280 | 31.081 | 28.767 | 31.697 |
LFMB5D 1st step | ||||||||||||
?=10 | 35.269 | 33.123 | 33.287 | 34.717 | 34.300 | 34.016 | 32.885 | 34.820 | 33.792 | 35.167 | 34.173 | 37.103 |
?=20 | 34.333 | 31.594 | 32.098 | 32.660 | 32.773 | 32.097 | 31.117 | 33.280 | 31.997 | 34.435 | 31.745 | 35.597 |
?=30 | 33.690 | 30.415 | 31.343 | 31.207 | 31.652 | 30.567 | 29.958 | 32.071 | 30.791 | 33.772 | 30.367 | 34.370 |
?=40 | 33.024 | 29.459 | 30.688 | 30.011 | 30.725 | 29.266 | 29.080 | 31.089 | 29.851 | 33.073 | 29.393 | 33.259 |
?=50 | 32.334 | 28.616 | 30.037 | 28.993 | 29.881 | 28.221 | 28.366 | 30.166 | 29.055 | 32.290 | 28.577 | 32.396 |
LFMB5D 2nd step | ||||||||||||
?=10 | 35.854 | 36.275 | 35.263 | 36.868 | 36.767 | 36.813 | 34.941 | 37.406 | 35.661 | 36.062 | 36.213 | 39.910 |
?=20 | 34.791 | 33.577 | 33.612 | 33.954 | 34.538 | 33.690 | 32.334 | 34.958 | 33.423 | 35.200 | 32.942 | 37.549 |
?=30 | 34.216 | 32.002 | 32.736 | 32.295 | 33.192 | 31.779 | 30.873 | 33.482 | 31.975 | 34.565 | 31.272 | 36.030 |
?=40 | 33.667 | 30.851 | 32.028 | 31.037 | 32.156 | 30.352 | 29.872 | 32.382 | 30.910 | 33.938 | 30.143 | 34.773 |
?=50 | 33.086 | 29.908 | 31.390 | 30.027 | 31.292 | 29.245 | 29.106 | 31.463 | 30.040 | 33.267 | 29.246 | 33.769 |
Amethyst | Bracelet | Chess | Eucalyptus Flowers | Jelly beans | Lego Bulldozer | Lego Knights | Lego Truck | Tarot Cards and Crystal Ball (Large Angle) | Tarot Cards and Crystal Ball (Small Angle) | The Stanford Bunny | Treasure Chest | |
HF4D | ||||||||||||
?=10 | 31.374 | 30.464 | 31.362 | 29.466 | 31.330 | 29.850 | 30.352 | 31.315 | 29.080 | 31.050 | 31.361 | 29.913 |
?=20 | 25.862 | 25.201 | 25.816 | 25.175 | 25.468 | 25.243 | 25.210 | 26.000 | 24.738 | 25.430 | 25.578 | 25.463 |
?=30 | 22.543 | 21.864 | 22.576 | 22.154 | 22.035 | 22.181 | 21.966 | 22.813 | 21.660 | 22.088 | 22.189 | 22.492 |
?=40 | 20.200 | 19.508 | 20.284 | 19.908 | 19.671 | 19.967 | 19.646 | 20.570 | 19.396 | 19.756 | 19.818 | 20.325 |
?=50 | 18.406 | 17.735 | 18.525 | 18.143 | 17.901 | 18.259 | 17.867 | 18.845 | 17.650 | 17.989 | 18.017 | 18.633 |
BM3D | ||||||||||||
?=10 | 37.549 | 38.438 | 40.829 | 35.177 | 44.865 | 37.429 | 40.135 | 38.394 | 38.154 | 37.914 | 39.814 | 36.962 |
?=20 | 33.964 | 34.565 | 37.542 | 31.246 | 41.867 | 33.814 | 36.666 | 34.917 | 34.578 | 34.267 | 36.744 | 33.047 |
?=30 | 31.925 | 32.290 | 35.398 | 29.093 | 39.696 | 31.680 | 34.489 | 32.796 | 32.445 | 32.099 | 34.788 | 30.816 |
?=40 | 30.518 | 30.645 | 33.777 | 27.600 | 37.985 | 30.156 | 32.872 | 31.259 | 30.884 | 30.511 | 33.320 | 29.195 |
?=50 | 29.422 | 29.271 | 32.412 | 26.354 | 36.548 | 28.934 | 31.566 | 30.037 | 29.586 | 29.175 | 32.137 | 27.764 |
BM3D EPI | ||||||||||||
?=10 | 38.915 | 38.879 | 41.029 | 35.688 | 43.871 | 37.076 | 38.430 | 39.171 | 36.684 | 37.955 | 41.338 | 37.703 |
?=20 | 36.234 | 35.472 | 38.871 | 31.760 | 41.685 | 33.122 | 34.473 | 36.180 | 32.283 | 34.970 | 39.329 | 33.358 |
?=30 | 34.481 | 32.174 | 37.207 | 29.398 | 40.229 | 30.714 | 32.317 | 33.970 | 29.493 | 33.040 | 37.754 | 31.090 |
?=40 | 31.200 | 31.430 | 35.894 | 27.748 | 39.080 | 29.031 | 31.015 | 32.307 | 27.596 | 31.557 | 36.484 | 29.412 |
?=50 | 29.875 | 28.992 | 34.800 | 26.529 | 38.127 | 27.814 | 30.117 | 31.003 | 26.232 | 30.331 | 35.305 | 27.997 |
VBM4D | ||||||||||||
?=10 | 38.561 | 38.997 | 41.004 | 36.531 | 43.941 | 38.334 | 40.253 | 39.164 | 37.906 | 38.433 | 40.225 | 37.882 |
?=20 | 34.974 | 35.156 | 37.391 | 32.917 | 40.098 | 34.558 | 36.525 | 35.580 | 34.190 | 34.830 | 36.736 | 34.094 |
?=30 | 32.657 | 32.748 | 34.921 | 30.679 | 37.411 | 32.142 | 34.066 | 33.211 | 31.893 | 32.551 | 34.476 | 31.792 |
?=40 | 30.944 | 30.959 | 33.041 | 29.020 | 35.341 | 30.370 | 32.212 | 31.440 | 30.192 | 30.853 | 32.763 | 30.099 |
?=50 | 29.592 | 29.526 | 31.535 | 27.704 | 33.667 | 28.980 | 30.729 | 30.035 | 28.834 | 29.494 | 31.384 | 28.755 |
VBM4D EPI | ||||||||||||
?=10 | 38.417 | 38.139 | 41.133 | 34.468 | 44.278 | 36.959 | 39.320 | 38.809 | 36.960 | 37.853 | 40.927 | 37.097 |
?=20 | 35.772 | 34.897 | 38.521 | 31.829 | 41.131 | 33.521 | 36.227 | 35.460 | 33.350 | 35.077 | 38.127 | 33.340 |
?=30 | 34.016 | 32.810 | 36.608 | 29.999 | 38.950 | 31.355 | 34.077 | 33.236 | 31.105 | 33.233 | 36.245 | 31.061 |
?=40 | 32.708 | 31.263 | 35.095 | 28.634 | 37.267 | 29.785 | 32.443 | 31.556 | 29.496 | 31.851 | 34.832 | 29.384 |
?=50 | 31.668 | 30.033 | 33.864 | 27.561 | 35.901 | 28.556 | 31.175 | 30.202 | 28.241 | 30.749 | 33.706 | 28.054 |
LFMB5D 1st step | ||||||||||||
?=10 | 38.847 | 38.907 | 40.797 | 36.710 | 43.926 | 38.630 | 40.351 | 39.385 | 37.043 | 38.432 | 40.826 | 38.229 |
?=20 | 35.529 | 35.037 | 37.415 | 33.399 | 40.399 | 35.026 | 36.972 | 35.873 | 33.355 | 35.228 | 36.787 | 34.786 |
?=30 | 33.190 | 32.515 | 35.162 | 31.306 | 37.294 | 32.624 | 34.781 | 33.479 | 30.940 | 32.856 | 33.911 | 32.469 |
?=40 | 31.368 | 30.857 | 33.466 | 29.658 | 34.400 | 30.828 | 33.038 | 31.664 | 29.122 | 30.773 | 32.078 | 30.702 |
?=50 | 29.862 | 29.587 | 32.046 | 28.174 | 32.257 | 29.321 | 31.588 | 30.176 | 27.749 | 29.021 | 30.743 | 29.129 |
LFMB5D 2nd step | ||||||||||||
?=10 | 39.593 | 39.884 | 42.158 | 37.263 | 45.636 | 39.603 | 41.598 | 40.357 | 38.354 | 39.344 | 41.881 | 39.003 |
?=20 | 37.020 | 36.988 | 40.045 | 34.332 | 43.826 | 36.762 | 38.936 | 37.852 | 35.371 | 36.673 | 39.383 | 36.071 |
?=30 | 35.257 | 35.305 | 38.437 | 32.575 | 42.281 | 34.878 | 37.167 | 36.062 | 33.618 | 35.007 | 37.560 | 34.227 |
?=40 | 33.874 | 34.077 | 37.056 | 31.286 | 41.016 | 33.438 | 35.761 | 34.592 | 32.301 | 33.708 | 36.090 | 32.858 |
?=50 | 32.685 | 33.020 | 35.851 | 30.142 | 39.920 | 32.155 | 34.598 | 33.343 | 31.269 | 32.615 | 34.837 | 31.693 |